Limits and potential of Deep Generative AI Models in Engineering
Deep generative models (DGMs) like ChatGPT are making waves with their remarkable ability to produce poetic lines, complete symphonies, and craft visuals based on vast volumes of previous works.
Yet, a fresh perspective from MIT engineers suggests these AI behemoths might fall short in genuinely pioneering engineering tasks.
The Core Challenge
According to Lyle Regenwetter, a mechanical engineering graduate student at MIT and the lead author of the study, “While DGMs excel at emulating datasets, true engineering often demands designs that diverge from existing ones.”
Collaborator Faez Ahmed, an assistant professor at MIT, further elaborated, “The success of many of these models is rooted in the statistical resemblance of their outputs to past examples. Yet, for true innovation, uniqueness is paramount.”
Key Findings and Collaborative Effort
Their research, published online and set for the December print edition of Computer Aided Design, sheds light on the inherent challenges DGMs face in engineering design tasks. For instance, while analysing bicycle frame design, they found that traditional DGMs often reproduced frames echoing prior designs, which may not necessarily meet rigorous engineering standards.
The study is the fruit of a partnership between experts at the MIT-IBM Watson AI Lab and MIT’s DeCoDe Lab, including notable contributions from Akash Srivastava and Dan Gutreund.
Deep Dive into DGM Capabilities
Delving deeper, DGMs are celebrated for their unparalleled data processing prowess. ChatGPT, for instance, belongs to a subset of DGMs known as large language models (LLMs) which blend natural language processing for more organic interactions. Simultaneously, other models, such as DALL-E and Stable Diffusion, cater to image generation.
Relevance in Engineering Domains
Despite their growing adoption in various engineering sectors, DGMs largely replicate current designs. Regenwetter points out a critical oversight: “Designers utilizing DGMs often overlook the crucial step of tailoring the model’s objectives to the design’s unique requirements.”
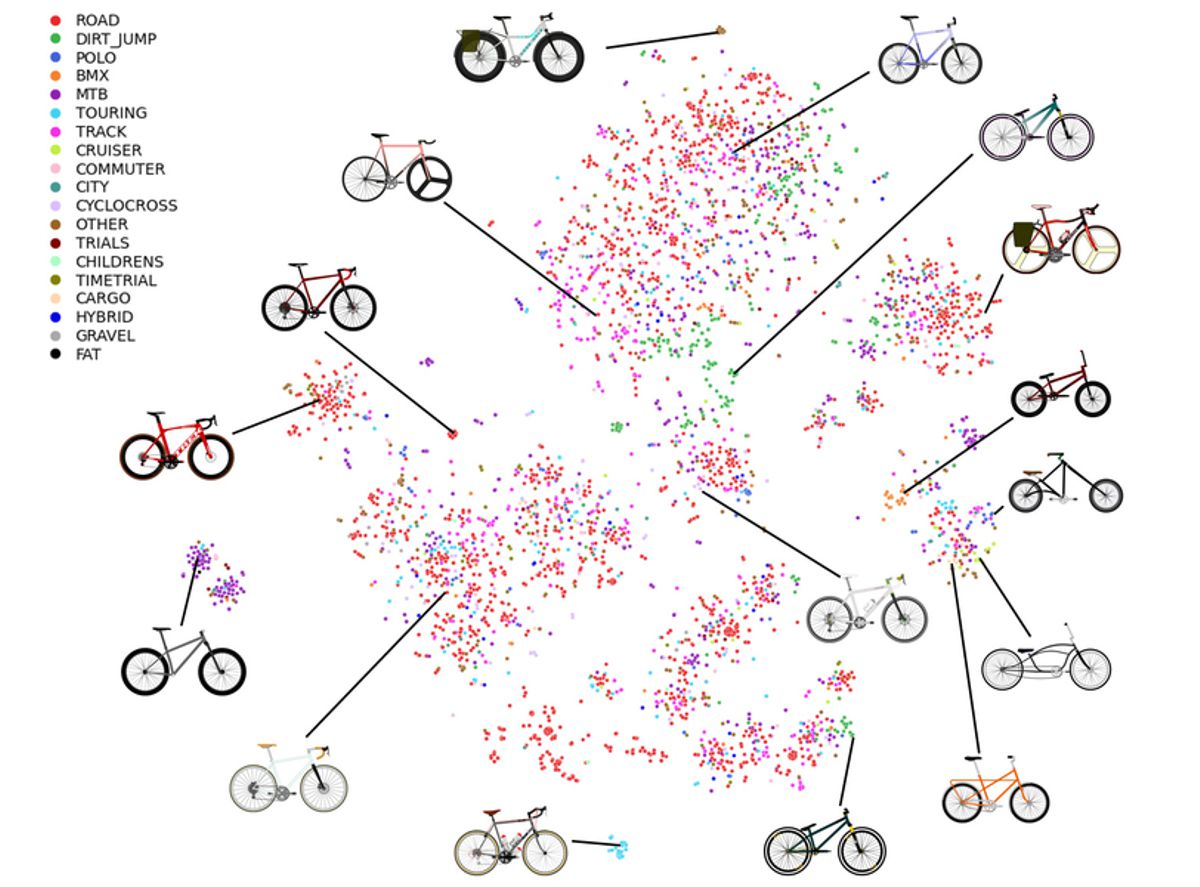
Bicycle Frame Design: A Case Study
The researchers elaborated on these challenges using bicycle frame designs as a case in point.
They noted that a model trained on countless existing frames might misinterpret the performance of frames with similar dimensions. Such an oversight could compromise structural integrity.
Testing and Results
The team’s rigorous tests included conventional models like generative adversarial networks (GANs) and specialized DGMs designed for engineering tasks. While GANs crafted designs reminiscent of existing frames without significant improvements, the specialized DGMs yielded better results.
One such DGM, crafted by Regenwetter to develop novel geometric structures, emerged as the most promising, delivering top-tier and feasible designs.
Conclusion and Future Outlook
Ahmed concluded: “Transcending mere statistical similarity allows a model to devise designs superior to existing ones. This serves as a testament to AI’s potential when deliberately trained for a specific design task.”
He envisions a future where DGMs, fine-tuned for performance, design constraints, and novelty, could revolutionize sectors like molecular design and civil infrastructure.
Through their study, the team aspires to pave the way for novel generative AI applications beyond mere multimedia.